Validating Your AI Startup Idea Before Development
Last Updated: May 21st 2025
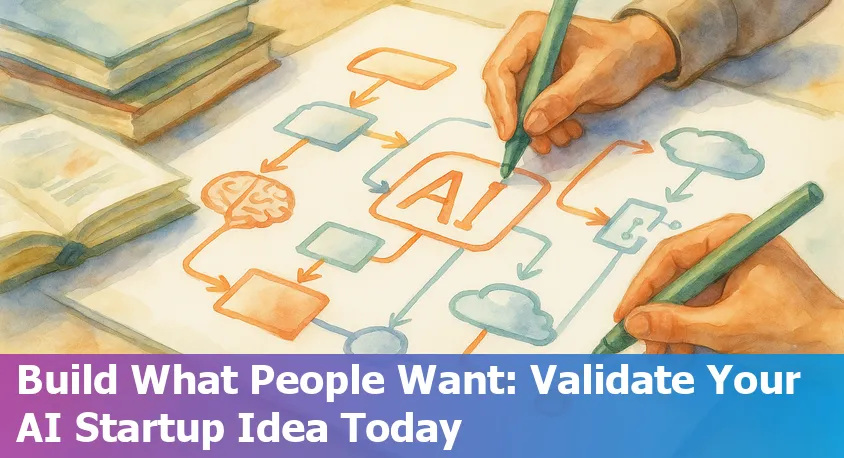
Too Long; Didn't Read:
Validating your AI startup idea before development is essential - 90% of startups fail, often due to lack of market fit. Use MVPs, data-driven testing, and AI-powered tools for user feedback and market analysis. Structured validation cycles and proven frameworks can significantly increase your chances of achieving product-market fit and long-term success.
Validating your AI startup idea before committing significant resources is a critical step in beating the odds - nearly 90% of startups fail, often because their solutions don't address real-world needs or lack market fit.
As highlighted by Harvard Business Review's analysis of common reasons why startups fail, “most start-ups don't succeed: More than two-thirds…never deliver a positive return to investors,” emphasizing the value of early validation efforts to reduce risk and confirm your idea actually solves a meaningful problem.
Practical validation means going beyond assumption and building simple MVPs, landing pages, or even mockups to test whether real users will engage, inquire, or pay before full development, as described in proven validation techniques from successful founders.
As Drexel University notes, idea validation “proves that your business solves a problem, can fill a need and can be marketed to consumers” - giving you crucial confidence to move forward (why validating a startup idea is so important).
By dedicating time to validate early and methodically, aspiring entrepreneurs dramatically boost their chances of building an AI business with genuine impact and staying power.
Table of Contents
- Step 1: Understanding Your Target Market and Problem Space
- Step 2: Testing Core Assumptions with Data and User Feedback
- Step 3: Prototyping and Building Low-Cost MVPs
- Step 4: Accelerating Validation Using AI-Powered Tools
- Step 5: Iteration, Refinement, and Continuous Validation
- Frameworks and Best Practices for AI Startup Validation
- Real-World Examples: Successful AI Startup Validation Stories
- Conclusion: Key Takeaways and Next Steps for Aspiring AI Founders
- Frequently Asked Questions
Check out next:
Find out how the cost to start an AI company in 2025 has plummeted, letting solo founders launch startups with minimal investment.
Step 1: Understanding Your Target Market and Problem Space
(Up)Before diving into AI product development, founders must rigorously define both the target market and the specific problem they intend to solve. Effective market validation starts with understanding who your ideal customers are, what pain points they face, and the magnitude of potential demand - vital steps for prioritizing features, aligning go-to-market strategy, and demonstrating viability to investors.
As highlighted in the F22 Labs market sizing framework, breaking down your audience into Total Addressable Market (TAM), Serviceable Available Market (SAM), and Serviceable Obtainable Market (SOM) provides a clear, structured view of opportunity.
For example, a startup offering AI video dubbing can estimate its TAM by calculating the global demand, then refine SAM and SOM by narrowing in on language, region, and realistic penetration rates.
A bottom-up approach - multiplying the number of target customers by average annual spend - not only delivers more credible projections but is also the preferred method among VCs, according to insights from Pear VC's Market Sizing Guide.
The AI sector itself is rapidly scaling, with 2025's global artificial intelligence market projected at $244.22 billion and expected to surpass $1 trillion by 2031 at a CAGR exceeding 26% (Statista AI market forecast).
Accurately sizing your market reduces the risk of targeting oversaturated or tiny niches and empowers smarter product and resource decisions. See the example below for how TAM, SAM, and SOM can break down an AI solution's market opportunity:
Market Level | Number of Customers | Price per User (Annual) | Market Size |
---|---|---|---|
TAM (Total Market) | 5M creators worldwide | $600 | $3B |
SAM (Reachable) | 1M creators (language-focused) | $600 | $600M |
SOM (Capturable) | 50,000 customers | $600 | $30M |
“Estimating market size is essential to validate demand, refine audience targeting, and set realistic growth expectations prior to full-scale MVP development.” - F22 Labs
Step 2: Testing Core Assumptions with Data and User Feedback
(Up)Testing your AI startup's core assumptions relies on a disciplined, data-driven approach - ensuring you focus on what truly works for your users and market. Begin by formulating precise, testable hypotheses about the problem, solution, target audience, and value proposition you believe your AI product can deliver.
Each hypothesis should be anchored in measurable outcomes, not mere intuition - for instance: "If we automate user onboarding with AI, then sign-up completion rates will increase by 20% within one month." Testing these assertions involves structured cycles: conduct surveys and in-depth user interviews to understand pain points and validate demand; deploy prototypes, A/B tests, or even 'fake door' landing pages to observe real-world interest and collect actionable data.
Embedding AI tools into this process can dramatically accelerate and sharpen your research - for example, platforms like quantilope, Speak, and Hotjar offer automated survey analysis, sentiment detection, and behavioral insights, while AI-powered research matrices can synthesize qualitative feedback at speed.
As highlighted in one industry guide, "Agility and adaptability in this cycle save resources and bring ideas closer to success."
Refuting a hypothesis is as important as confirming it.
To help founders compare methods, here is a simple table of commonly used validation techniques:
Technique | Purpose | Tools/Examples |
---|---|---|
User Interviews & Surveys | Gather direct feedback on needs and problems | Quantilope, Speak, traditional survey tools |
A/B & Usability Testing | Assess product changes by measuring user behavior | Hotjar, VWO, in-app analytics |
Prototypes & Fake Door Tests | Measure actual demand and intent before full build | Landing pages, clickable demos |
Iterate based on what you learn - follow the data, not assumptions - and remember that validation is an ongoing process that sharpens product-market fit and maximizes your chances of startup success.
For further insights on using advanced AI tools to supercharge market research and user feedback analysis, see this overview of startup validation case studies using AI, a detailed guide on the best AI market research tools and their features, and a practical breakdown on startup hypothesis testing frameworks.
Step 3: Prototyping and Building Low-Cost MVPs
(Up)When building a low-cost MVP for your AI startup, strategic planning and disciplined resource allocation are key to maximizing validation while minimizing risk.
Emphasize core generative AI features and use frameworks like the MoSCoW method to avoid feature creep - prioritizing only the functionalities that are essential for real-world testing and scalability.
A scalable architecture from the outset is vital; cloud-native resources such as AWS Sagemaker or Google Cloud AI Platform can significantly improve flexibility while controlling costs.
According to a detailed Gen AI MVP guide for 2025, the most common pitfall is treating AI MVPs like traditional software, which often leads to costly rewrites as model retraining and data integration needs grow.
Depending on your development approach and complexity, costs can range from $5,000 for simple prototypes with freelancers, to $35,000 or more with agencies specializing in rapid, scalable MVP builds.
The following table summarizes cost ranges for common MVP development strategies:
Approach | Cost Range | Best For |
---|---|---|
Freelancers | $4,000–$15,000 | Simple MVPs, quick iterations |
Outsourced Agencies | $10,000–$35,000 | Mid-complexity projects |
In-House Teams | $25,000–$150,000 | Scalable, long-term builds |
Incorporate rapid prototyping, continuous feedback loops, and agile methodologies to ensure that even with limited funds, your MVP delivers validated learning from target users.
Efficient use of open-source frameworks and no-code tools further compresses development time and cost, as highlighted in the guide to building MVPs fast and cheap.
Above all, focus on measurable impact:
“Focus on minimal yet functional products validated by user feedback. Start small for flexibility and adapt based on real market needs.”
- from Maxiom's budget MVP roadmap
Step 4: Accelerating Validation Using AI-Powered Tools
(Up)Accelerating the validation of your AI startup idea is now more accessible than ever with advanced AI-powered tools that streamline market research, competitive analysis, and user feedback.
Platforms like Validator AI for startup idea validation offer real-time value proposition writing, instant market analysis, and startup scoring, allowing founders to quickly assess launch readiness and receive actionable next steps - features traditionally reserved for expensive consultancy sessions.
These tools combine data automation, customer segmentation, and predictive analytics to help identify demand and refine core assumptions, ensuring your product aligns with genuine market needs.
As highlighted in the AI Market Research Review for Startups, leveraging AI not only expedites validation but also enhances strategic decision-making by identifying target audiences and automating competitor analysis.
Here's a quick comparison of AI-powered versus traditional advisor features:
Feature | Validator AI | Traditional Mentors |
---|---|---|
24/7 Availability | (Appointment Needed) | |
Real-time Market & Competition Analysis | ||
Personalized Next Steps & Assets | ||
Cost-Effective (from $0 for basic use) | (Often $100–$500+ per session) |
As the landscape grows, other AI-driven validation tools like SparkRockets and Fe/male Switch enable entrepreneurs to build personalized business roadmaps and refine strategies in minutes (Top 10 Startup Idea Validation Tools 2025).
These advancements ensure even first-time founders can efficiently iterate their ideas and gain the clarity needed to launch with confidence.
Step 5: Iteration, Refinement, and Continuous Validation
(Up)Step 5 in validating your AI startup idea emphasizes the continuous loop of iteration, refinement, and validation - where user feedback directly shapes your product roadmap.
Establishing a structured user feedback loop is critical: gather insights via regular surveys, interviews, in-app widgets, and social listening, then organize this data by themes, priority, and sentiment for actionable planning.
The most successful founders rapidly implement changes, assess their impact via core metrics such as Net Promoter Score (NPS), Customer Satisfaction Score (CSAT), and Customer Effort Score (CES), and openly communicate updates to users, reinforcing trust and encouraging ongoing engagement.
Leveraging modern tools like Typeform, UserTesting, and Userback as highlighted in this practical guide to building a user feedback loop for startups and advanced analysis platforms recommended by experts in user feedback analysis methodologies streamlines this process.
AI-powered platforms, such as ChatGPT and Microsoft Copilot, now enable teams to condense days of manual data review into actionable strategies in mere hours, making iteration both efficient and scalable.
As the team from Part-Time Founders explains, “AI tools like ChatGPT and Microsoft Copilot make it easier than ever to turn raw data into actionable insights”
“Customer feedback is the secret fertilizer that can transform your business from a patchy lawn into a lush, thriving oasis.”
For a comparison of top feedback tools to keep your validation process agile, see the table below:
Tool | Best For | Key Features |
---|---|---|
Typeform | Surveys & Forms | Custom design, analytics |
UserTesting | User Research | First-person feedback, video, journey mapping |
Userback | Visual Feedback | In-app capture, bug reports, integrations |
To maximize your startup's chances of product-market fit, maintain regular feedback cycles, prioritize high-impact insights, apply changes strategically, and always communicate progress with your users - turning customer voices into your most valuable scaling asset.
Discover more about harnessing user input for rapid growth in this customer feedback analysis guide.
Frameworks and Best Practices for AI Startup Validation
(Up)Effectively validating your AI startup idea before development hinges on employing proven frameworks and industry best practices - chief among them, the Lean Startup Methodology.
This approach emphasizes rapidly testing assumptions through cycles of building minimum viable products (MVPs), measuring results with real customer data, and learning whether to pivot or persevere, thus minimizing wasted resources and maximizing validated insights (Lean Startup principles overview by Eric Ries).
Central to this process is Lean Validation, which systematically tests the desirability, feasibility, viability, and responsibility of a business idea before significant investment.
Lean Validation's stepwise methodology uses low-cost experiments, user interviews, MVP testing, and ideally, a “validation canvas” tool to structure assumptions, experiments, and outcomes, ensuring decision-making is backed by data rather than gut instinct (Bundl's Lean Validation Guide).
For AI-driven startups, integrating AI tools into research, prototype development, and feedback analysis can exponentially speed up these validation cycles - enabling startups to build and iterate faster, test business hypotheses across synthetic populations, and glean actionable metrics to further de-risk the innovation journey (How AI is Revolutionizing Lean Startup Validation).
In sum, using well-established frameworks, rigorous customer validation techniques, and the latest AI-powered tools dramatically raises your odds of building a product the market genuinely wants, while minimizing costly missteps along the way.
Real-World Examples: Successful AI Startup Validation Stories
(Up)Examining real-world case studies is invaluable for understanding how successful AI startups validate their ideas before scaling. Startups such as Vanta, Cocoon, and Good Dog exemplify a diversity of approaches.
For instance, Vanta validated demand for automated SOC 2 compliance by rapidly building a no-code MVP and gathering strong early feedback, while Cocoon leveraged interactive prototypes to sign their first customers before launching fully, and Good Dog performed in-depth interviews alongside "sacrificial concept" mockups to ensure marketplace fit.
Other founders, like those at Snackpass and Rec Room, tested value and virality with tightly focused, local launches and manual outreach before broadening their platforms.
Across these stories, certain best practices emerge: grounding assumptions in direct user conversations, using simple prototypes, and being unafraid to pivot or double down based on genuine market signals.
As highlighted in a deep-dive by Todd Jackson on startup idea validation, founders who methodically draw on customer pain, prototype iteratively, and watch for strong ‘pull' from their target users are more likely to build not just a tool but a business with true product-market fit.
To speed up modern validation cycles, AI-powered platforms like GoZigzag now help entrepreneurs generate lean canvases, customer validation questions, and storytelling resources from a single sentence, as seen in their adoption by founders across 115 countries (learn more from the SXSW 2024 talk on AI-powered idea validation).
These advancements reduce time-to-validation from months to days, but regardless of the tools, all case studies reinforce that the combination of systematized learning, authentic customer engagement, and disciplined interpretation of signals underpins successful AI startup validation.
For a clear framework and side-by-side look at both traditional and AI-enhanced tools - plus practical tips on testing assumptions and interpreting market demand - review this practical guide on how to validate startup ideas with AI.
Conclusion: Key Takeaways and Next Steps for Aspiring AI Founders
(Up)Validating your AI startup idea prior to development is more than a one-off task - it's an ongoing, iterative process that dramatically increases your chance of achieving product-market fit.
Leading founders and experts emphasize the role of market research, direct user engagement, and rapid prototyping to test core assumptions, refine offerings, and confirm real demand.
As highlighted by real-world success stories, the most effective approaches are systematic: identify unique user pain points, launch MVPs or solution prototypes, gauge authentic willingness to pay, and use feedback to guide each iteration.
Leveraging modern AI tools can further accelerate the validation cycle - shaving weeks off research, surfacing market gaps, and helping founders make data-driven pivots with confidence.
"Validation involves progressing from market analysis to user engagement, prototype testing, and iterative feedback."
The key takeaways for aspiring AI founders are: 1) never skip validation; 2) structure your process using proven frameworks and data-driven tools; and 3) embrace feedback and learning as central to your growth trajectory.
For those ready to put these insights into action, Nucamp's Solo AI Tech Entrepreneur Bootcamp offers targeted education on startup validation, business modeling, and lean MVP launch strategies.
Dive deeper into step-by-step frameworks with this insightful guide on how to validate startup ideas with AI, or explore practical, field-tested advice from leading startup founders via Lenny's Newsletter's validation case studies.
Put simply, rigorous validation is not just a best practice - it's a strategic advantage that saves time and capital while positioning your startup for long-term success.
Frequently Asked Questions
(Up)Why is it important to validate my AI startup idea before development?
Validating your AI startup idea beforehand dramatically reduces your risk of failure by ensuring your solution addresses a real-world need and has market fit. Without validation, you risk building something nobody wants - statistics show that nearly 90% of startups fail, often for this reason. Early validation helps you confirm demand, optimize your resources, and increase your chances of long-term success.
What are the main steps to validate an AI startup idea?
The main steps include: 1) Understanding your target market and the problem you're solving, 2) Testing your core assumptions with user feedback and data, 3) Building low-cost prototypes or MVPs to observe real user interest, 4) Accelerating validation with AI-powered research and feedback tools, and 5) Iterating based on insights through continuous feedback and refinement cycles.
How do I estimate the market size for my AI startup?
Estimate your market size using TAM (Total Addressable Market), SAM (Serviceable Available Market), and SOM (Serviceable Obtainable Market). Start with the broadest potential customer base, then narrow it down to those you can realistically reach and convert. Use a bottom-up approach - multiply the number of likely customers by expected annual spend for each market segment for more credible projections.
What tools and methods can I use for rapid validation of my AI startup idea?
You can use user interviews, surveys, A/B tests, 'fake door' landing pages, and simple prototypes to gauge real demand. AI-powered validation platforms like quantilope, Speak, Hotjar, and startup validation AIs offer automated research, instant market analysis, and actionable insights, allowing for much faster and more affordable validation than traditional mentorship and consulting.
What frameworks or best practices should I follow when validating my AI startup?
Adopt frameworks like Lean Startup and Lean Validation, which focus on low-cost experiments, rapid MVP development, and data-driven decision-making. Systematically test desirability, feasibility, and viability using structured assumptions and feedback loops. Leverage modern AI tools to accelerate cycles and ensure your decisions are informed by real user data - not just gut instinct.
You may be interested in the following topics as well:
Transform your development process by adopting secure coding best practices right from day one.
Take actionable steps toward optimizing your AI infrastructure for cost savings and operational efficiency from day one.
Stay ahead with essential insights on privacy compliance for outreach automation as regulations evolve for startups.
Avoid expensive mistakes by reading up on common MVP pitfalls in AI startups that often derail promising projects.
Boost security and efficiency for your AI startup using Nextcloud private team storage for all your project files and shared resources.
Streamline your AI pipelines and automate complex workflows using Haystack workflow orchestration without worrying about vendor lock-in.
Boost decision-making accuracy and reliability by incorporating human-in-the-loop practices as part of your AI development workflow.
Acknowledge the challenges unique to solo AI founders and learn how to tackle them head-on.
Ludo Fourrage
Founder and CEO
Ludovic (Ludo) Fourrage is an education industry veteran, named in 2017 as a Learning Technology Leader by Training Magazine. Before founding Nucamp, Ludo spent 18 years at Microsoft where he led innovation in the learning space. As the Senior Director of Digital Learning at this same company, Ludo led the development of the first of its kind 'YouTube for the Enterprise'. More recently, he delivered one of the most successful Corporate MOOC programs in partnership with top business schools and consulting organizations, i.e. INSEAD, Wharton, London Business School, and Accenture, to name a few. With the belief that the right education for everyone is an achievable goal, Ludo leads the nucamp team in the quest to make quality education accessible