Reducing Operational Costs Through Efficient Self-Hosting for AI Startups
Last Updated: May 21st 2025
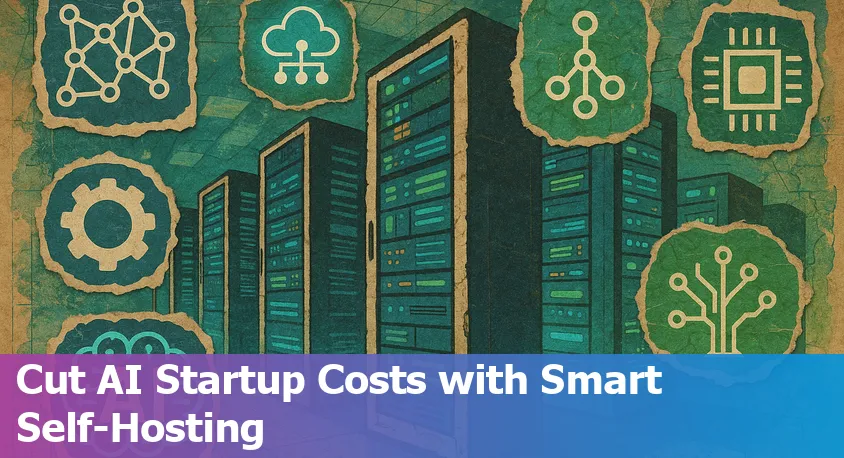
Too Long; Didn't Read:
Efficient self-hosting enables AI startups to reduce operational costs by up to 50%, maintain data privacy, and avoid unpredictable cloud fees. While upfront hardware investment may exceed $10,000, long-term savings, compliance with GDPR and HIPAA, and customizable AI performance make self-hosting a strategic choice for scalable, cost-effective AI deployment.
The rapid growth of artificial intelligence in 2025 presents a critical cost challenge for startups looking to innovate without breaking the bank. Developing and deploying AI solutions involves a wide spectrum of expenses, from data preparation and compute infrastructure to ongoing maintenance and regulatory compliance.
For example, building an AI system can cost anywhere from $10,000 for a simple chatbot to over $300,000 for a sophisticated, enterprise-grade platform, with ongoing infrastructure and management sometimes exceeding $5,000 per month for mid-sized solutions (comprehensive AI cost breakdown for 2025).
Beyond upfront development, unpredictable cloud compute costs, hidden expenses from data handling, and lower gross margins compared to traditional SaaS products squeeze profitability and make financial planning complex for early-stage ventures (AI pricing strategies and margins in 2025).
As a result, efficient cost management - including exploring options like self-hosting, open-source tools, and phased rollouts - has become essential for AI founders determined to turn technical breakthroughs into sustainable business models (key trends and pitfalls in AI budgeting).
Table of Contents
- What is Self-Hosting in AI?
- Comparing Costs: Self-Hosting vs. Cloud AI Services
- Benefits of Efficient Self-Hosting for AI Startups
- Common Pitfalls and Hidden Costs to Watch Out For
- Best Practices: How AI Startups Can Optimize Self-Hosting
- When Should AI Startups Choose Cloud Over Self-Hosting?
- Real-World Success Stories: Savings from Self-Hosting AI
- Conclusion: The Path to Cost-Efficient, Scalable AI for Startups
- Frequently Asked Questions
Check out next:
Steer clear of common mistakes by understanding avoiding solo founder pitfalls in AI startups and adopting the right mindset for success.
What is Self-Hosting in AI?
(Up)Self-hosting in AI refers to deploying and managing AI models, applications, or services on infrastructure you directly own or control - whether that's on-premises hardware, private cloud, or a hybrid environment - rather than relying on third-party, cloud-based providers.
This approach grants organizations full autonomy over data, algorithms, and system configurations, making it especially attractive to businesses with stringent privacy, regulatory, or customization requirements.
As Lizard Global's guide to self-hosted AI solutions notes, self-hosting offers unparalleled data control, compliance with regulations like GDPR and HIPAA, and more predictable long-term costs.
It also allows for deeper model customization and performance tuning, but requires significant initial investment in hardware, software, and engineering expertise.
According to TechGDPR's analysis on self-hosting AI for privacy compliance and cost efficiency, self-hosting ensures that sensitive data never leaves your servers, reducing both breach risks and reliance on fluctuating subscription fees or external policy changes.
In essence, as summarized by Ente's beginner's guide to self-hosting, this model provides independence and cost efficiency while placing responsibility for setup, security, and ongoing maintenance squarely in your hands.
For AI startups, self-hosting is a strategic choice that balances operational control and scalability against the complexity and overhead of running your own stack.
Comparing Costs: Self-Hosting vs. Cloud AI Services
(Up)When evaluating operational costs for AI startups, the choice between self-hosting and cloud-based AI services is pivotal. Self-hosting requires a significant initial investment - such as acquiring an NVIDIA A100 GPU for around $10,000 - along with ongoing expenses for power, cooling, and maintenance.
While this approach empowers startups with unrivaled control and eliminates unpredictable API or token-based fees, it demands technical expertise and is often most cost-effective at higher usage volumes.
On the other hand, cloud AI services from major providers like AWS, Azure, and Google Cloud operate on a pay-as-you-go model, offering flexibility, no upfront hardware costs, and seamless scalability - with costs starting from as little as $872/month for a GPU instance (AWS g5.2xlarge) but potentially rising to $350,000 per year as user traffic scales according to a recent case study.
As summarized in a comprehensive comparison, cloud services are often more affordable for low or irregular traffic, while self-hosting pays off for startups with consistent, high-volume AI workloads that require customization and compliance see cost trade-offs here.
However, unexpected cloud expenses, such as storage and data transfer fees, can quickly escalate without vigilant cost management as detailed in this cloud AI cost guide.
The best choice depends on usage patterns, regulatory requirements, and available engineering skills, with many startups adopting a hybrid approach to balance flexibility, control, and long-term savings.
Benefits of Efficient Self-Hosting for AI Startups
(Up)Efficient self-hosting delivers AI startups a suite of compelling benefits, most notably robust data privacy, predictable cost structures, and unparalleled customization.
By operating AI models on-premises or on dedicated hardware, startups maintain full control over data flows - essential for compliance with regulations like GDPR and HIPAA - while also reducing data breach risks and avoiding reliance on third-party providers whose pricing and policies can change unexpectedly.
As highlighted by TechGDPR's analysis of self-hosted AI, eliminating recurring cloud subscription fees and fluctuating token costs leads to significant long-term savings, especially for steady AI workloads.
Furthermore, startups can fine-tune models to match specific business needs, offering flexibility that cloud solutions often lack. A recent industry review illustrates these advantages in practice:
Benefit | Description |
---|---|
Data Control & Privacy | All sensitive data remains on local infrastructure, simplifying compliance and protecting against external threats. |
Predictable Costs | Avoids unpredictable cloud and API billing, with costs limited mostly to upfront hardware and ongoing maintenance. |
Customization & Performance | Fine-tune models and infrastructure for low latency and operational efficiency, critical for real-time startups. |
As cybersecurity thought leader Nahla Davies notes,
“Self-hosted AI systems offer unmatched flexibility and customisation over the models used. Control over data handling, storage, and processing allows fine-tuning models.”
Ultimately, for startups seeking cost efficiency, data privacy, and the latitude to innovate, self-hosted AI platforms are an increasingly strategic choice, as further explored in this comprehensive guide to self-hosted AI solutions and a discussion on balancing innovation with security in self-hosted AI.
Common Pitfalls and Hidden Costs to Watch Out For
(Up)While efficient self-hosting promises autonomy and long-term savings, AI startups must be wary of several common pitfalls and hidden costs lurking beneath the surface.
Hardware investments - such as procuring top-tier GPUs, power management, and cooling infrastructure - represent significant upfront outlays and ongoing expenses, which can be easily underestimated by founders focused on cloud alternatives or pay-per-use APIs as outlined in this breakdown of real AI infrastructure costs.
Operational complexity compounds the challenge: onboarding new engineers, maintaining custom stacks, and scaling infrastructure to handle unpredictable surges in user demand all drive up the effort and total cost of ownership.
According to a large-scale enterprise survey, underestimated ongoing costs typically include system maintenance, staff training, repeated fine-tuning, and compliance with evolving legal and ethical frameworks - a detail many startups overlook as they chase rapid AI deployment.
As one CTO noted,
“It seems enterprises are moving quickly to generative AI but they may not be understanding the problems and challenges with it well enough. They're going to have to learn fast to compete at peak performance.”
Staff and training issues, data quality management, and opportunity costs from misaligned projects further erode early cost projections.
The table below summarizes areas where hidden costs commonly arise:
Cost Driver | Description |
---|---|
Hardware & Energy | Expensive GPUs, high energy/power demand, cooling requirements |
Staffing & Expertise | Hiring/retaining AI engineers, training, upskilling internal teams |
Data Management | Acquisition, cleaning, annotation, privacy compliance |
Operational Overhead | Infrastructure maintenance, DevOps, monitoring, downtime risk |
Legal & Ethical | Bias monitoring, liability protection, regulatory compliance |
For a deeper dive into surprising infrastructure hurdles and community-sourced lessons from self-hosting, explore the curated advice and cautionary stories shared by practitioners on r/selfhosted's discussion on AI models.
Additionally, understand how operational fine points - like redundancy and scaling - can cause self-hosting costs to match or even exceed those of managed services, as thoroughly compared in this VentureBeat analysis of OpenAI API versus DIY LLMs.
Best Practices: How AI Startups Can Optimize Self-Hosting
(Up)To maximize the cost efficiency and reliability of self-hosted AI infrastructure, startups should adopt a suite of best practices that address architecture, automation, observability, and resource management.
Begin by carefully evaluating and selecting AI tools that combine scalability, security, and developer flexibility for your needs - tools like MLflow streamline experiment tracking and deployment pipelines, while Kubernetes can automate containerization and horizontal scaling for both training and inference workloads (AI Infrastructure Guide for Provisioning and Managing ML Workloads).
Prioritize workload analysis and granular resource allocation to avoid idle GPU or CPU usage; workload management platforms such as Run:ai help visualize, schedule, and optimize jobs for maximum hardware utilization (Run:ai Managing Workloads and Resource Allocation for AI).
Once deployed, continuous observability is key - implement AI observability solutions to monitor performance, set up real-time alerts for failures, and ensure compliance, helping your stack stay robust as you scale (AI Observability Solutions for Reliable Performance in Self-Hosted AI Startups).
By automating pipeline management, right-sizing compute environments, and actively tracking system health, startups can achieve high efficiency, lower operational costs, and maintain agility throughout the machine learning lifecycle.
When Should AI Startups Choose Cloud Over Self-Hosting?
(Up)AI startups should opt for cloud-based solutions over self-hosting when they need rapid scalability, minimal upfront costs, and flexibility to handle unpredictable or low traffic workloads.
Leading cloud providers like Google Cloud, AWS, and Azure offer instant access to powerful AI models, seamless auto-scaling for demand spikes, and a pay-as-you-go pricing structure - key advantages for early-stage projects and startups lacking deep in-house infrastructure expertise.
As highlighted in in-depth comparisons of cloud AI vs. self-hosted LLMs, cloud AI is ideal for applications where usage is irregular, initial investment must stay low, or the goal is fast time-to-market.
Moreover, cloud-native AI services provide extensive libraries, global compliance options, and pre-integrated security, letting startups easily experiment and expand.
For example, Google Cloud powers hundreds of real-world AI deployments - from chat assistants in automotive and healthcare to scalable recommendation engines in retail - proving its strength in supporting high-growth, customer-facing solutions (see these generative AI use cases).
However, it's important to weigh possible trade-offs, such as hidden performance or cost challenges at scale and potential vendor lock-in, especially as business needs evolve (this analysis on cloud alternatives for AI startups).
In summary, cloud AI services offer startups a cost-effective, agile pathway to launch and refine MVPs, ideal when predictability, security, and fine-grained customization are not critical from the outset.
Real-World Success Stories: Savings from Self-Hosting AI
(Up)Numerous real-world success stories illustrate how efficient self-hosting strategies for AI can lead to substantial operational savings for startups and enterprises alike.
For example, TS Imagine leveraged self-hosted generative AI on Snowflake's Cortex platform, achieving a remarkable 30% reduction in costs and saving over 4,000 employee hours annually while maintaining high performance and scalability.
Their journey demonstrates that, when thoughtfully executed, self-hosting can outperform reliance on costly pretrained API solutions in both cost and efficiency Snowflake's real-world AI customer stories.
Similarly, early adopters profiled by Stellar.ai, ranging from global retailers to manufacturers, saw cost savings of 30–50%, increased productivity, and even $2M annually through AI-driven supply chain optimization, predictive maintenance, and customer service automation detailed case studies of AI-powered efficiency by Stellar.ai.
In more specialized applications, AI-powered translation solutions have been shown to reduce localization costs by up to 90%, enabling startups and SMEs to compete globally without breaking the bank Akvelon's industry report on AI-powered cost reduction.
These outcomes are not isolated; they underscore a broader trend - self-hosting AI, when optimized for organizational needs, translates directly into measurable cost savings, improved response times, and a tangible competitive advantage.
Conclusion: The Path to Cost-Efficient, Scalable AI for Startups
(Up)The path to cost-efficient, scalable AI for startups demands a strategic approach that balances long-term growth, control, and compliance needs. As compute and infrastructure costs remain a primary determinant of AI success - some startups allocate over 80% of capital to compute - self-hosting emerges as an attractive way to manage expenditure, retain data sovereignty, and meet stringent regulations like GDPR and HIPAA. Researchers note,
“What works for traditional apps became prohibitively expensive when applied to AI workloads”
and that
“It becomes unsustainable from a cost perspective” to scale real AI use cases in the public cloud
as highlighted by OpenMetal's analysis on AI startup alternatives to public cloud.
While cloud services offer invaluable flexibility and rapid scaling in early and unpredictable phases, the economics often shift as usage increases, introducing unpredictable costs and potential compliance hurdles.
By self-hosting, AI startups can benefit from predictable expenditures, enhanced privacy, and tailored performance: a Swiss study demonstrated self-hosted models can halve prediction costs with little to no loss in accuracy, according to TechGDPR.
However, it's essential to weigh upfront investments and the need for dedicated engineering expertise against the operational advantages, perhaps leveraging hybrid models or specialty clouds.
As the industry rapidly evolves, startups that master infrastructure efficiency and regulatory agility will hold the competitive edge - cultivating innovation on a foundation designed for scale.
For those ready to deepen their expertise in self-hosting, team building, and global SaaS scaling, Nucamp's 30-week Solo AI Tech Entrepreneur Bootcamp provides the structure and mentorship to launch market-ready AI ventures - learn more in this comprehensive bootcamp syllabus.
Frequently Asked Questions
(Up)What is self-hosting in AI and why should startups consider it?
Self-hosting in AI refers to running AI models, applications, or services on infrastructure directly owned or controlled by the startup - such as on-premises hardware, private cloud, or hybrid environments - instead of relying on third-party cloud providers. This gives startups full control over their data, privacy, compliance, and system customization. It is particularly attractive for startups with strict regulatory requirements or those aiming for predictable long-term costs and robust data sovereignty.
How do the costs of self-hosting compare to using cloud AI services?
Self-hosting requires significant upfront investment in hardware, such as high-end GPUs (e.g., NVIDIA A100 at ~$10,000 each), as well as ongoing power, maintenance, and staffing costs. These costs are offset by more predictable long-term expenses and no recurring cloud fees. In contrast, cloud AI services offer pay-as-you-go pricing, making them more affordable for low or variable traffic but potentially much more expensive as workloads scale (cloud GPU instances can start at around $872/month and rise substantially). The best choice depends on your usage patterns, compliance needs, and available technical expertise.
What are the main benefits of efficient self-hosting for AI startups?
Efficient self-hosting provides startups with strong data privacy, predictable and potentially lower operational costs, and the ability to deeply customize their AI systems for optimal performance. All sensitive data remains in-house, aiding in regulatory compliance (e.g., GDPR, HIPAA) and reducing breach risks. Avoiding unpredictable API and token-based billing from cloud providers enables more accurate financial planning, while the infrastructure can be tailored for specific business needs.
What are common hidden costs and pitfalls in self-hosting AI solutions?
Common hidden costs include underestimating expenses related to hardware acquisition, power and cooling, staff training, ongoing system maintenance, and compliance management. Operational complexity increases with onboarding new engineers and scaling infrastructure. Failing to account for the ongoing need for expert staff, data management, and legal compliance can result in higher total cost of ownership than anticipated, potentially matching or exceeding the cost of managed cloud services.
When should AI startups choose cloud services over self-hosting?
AI startups should favor cloud-based solutions in situations where rapid scalability, low initial investment, and flexibility for unpredictable or low traffic workloads are essential. Cloud platforms are ideal for projects that need fast deployment, experimentation, and minimal infrastructure management. However, as usage scales and compliance demands grow, the economic and operational advantages may shift towards self-hosting or hybrid models.
You may be interested in the following topics as well:
Take your business to the next level by building a data-driven retention system that leverages actionable insights from user analytics.
Discover why balancing automation with human touch is essential for an effective support strategy.
Maximize your concentration and productivity with proven deep work strategies built for solo founders in the AI space.
Understand the value of iteration and continuous validation to steer your AI product in the right direction.
Discover how marketing automation for AI startups can give your business a competitive edge from day one.
Explore why AWS-native AI coding tools are becoming essential for cloud-focused solo startups.
Take advantage of Stripe's currency conversion and FX APIs to offer seamless transactions in 135+ currencies.
Dive into the PostgreSQL extensibility for machine learning and see how it empowers rapid experimentation with AI models.
Ludo Fourrage
Founder and CEO
Ludovic (Ludo) Fourrage is an education industry veteran, named in 2017 as a Learning Technology Leader by Training Magazine. Before founding Nucamp, Ludo spent 18 years at Microsoft where he led innovation in the learning space. As the Senior Director of Digital Learning at this same company, Ludo led the development of the first of its kind 'YouTube for the Enterprise'. More recently, he delivered one of the most successful Corporate MOOC programs in partnership with top business schools and consulting organizations, i.e. INSEAD, Wharton, London Business School, and Accenture, to name a few. With the belief that the right education for everyone is an achievable goal, Ludo leads the nucamp team in the quest to make quality education accessible