Integrating Machine Learning into Your AI Startup Application
Last Updated: May 21st 2025
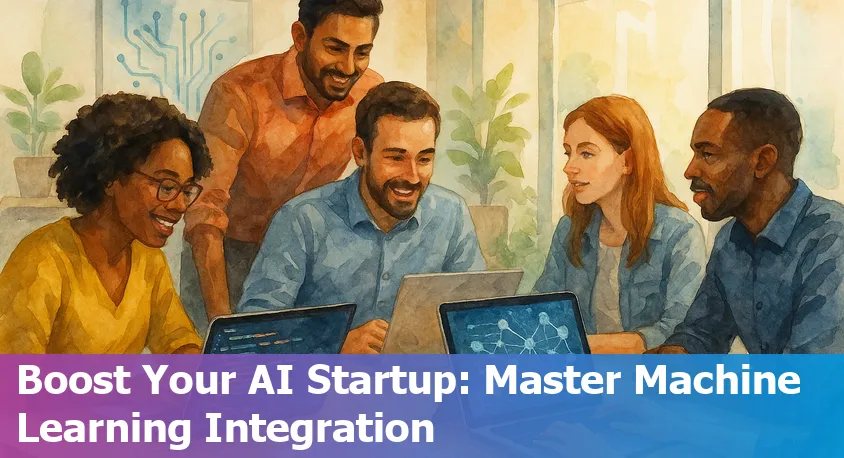
Too Long; Didn't Read:
Integrating machine learning into your AI startup boosts efficiency, automates tasks, and delivers personalized customer experiences, with real-world cases showing rapid scaling to 100,000+ monthly users. Key benefits include cost savings and data-driven insights, but common challenges involve data quality (65% cite issues) and integrating ML into workflows (44% face difficulty).
Integrating machine learning (ML) into your AI startup isn't just a trend - it's a strategic imperative for driving innovation, operational efficiency, and competitive advantage.
ML allows startups to automate routine tasks, deliver hyper-personalized customer experiences, and uncover data-driven insights previously out of reach through manual approaches.
As highlighted by industry experts,
“Machine learning is changing, or will change, every industry, and leaders need to understand the basic principles, the potential, and the limitations.” - Aleksander Madry, MIT Computer Science Professor
The practical impact is profound, from AI-powered chatbots and personalized recommendations to streamlined workflows and predictive analytics.
Early adoption enables startups to keep pace - if not outpace - established competitors by acting on trends faster and refining business strategies with real-time data.
Delaying ML integration can mean missed opportunities and a difficult scramble to catch up. For a deep dive into these transformative benefits, explore this comprehensive guide to AI and ML in startups, an industry-focused overview on AI and ML advantages for IT startups, and an expert explainer on why machine learning matters from MIT Sloan.
Table of Contents
- Key Benefits of Including Machine Learning in Your AI Startup Application
- Common Challenges for AI Startups Integrating Machine Learning
- Step-by-Step Guide: How Beginners Can Integrate Machine Learning
- Best Practices and Real-World Tips for AI Startup Success
- Advanced Strategies for Scaling Your ML-Powered AI Startup
- Frequently Asked Questions
Check out next:
Explore proven AI startup monetization strategies including SaaS models, marketplaces, and lucrative B2B offerings.
Key Benefits of Including Machine Learning in Your AI Startup Application
(Up)Integrating machine learning into your AI startup application delivers a myriad of tangible benefits - increasing operational efficiency, driving cost savings, and unlocking new avenues for innovation.
By automating repetitive processes such as data entry, customer support, and workflow management, ML allows your team to focus on creative, higher-value tasks, resulting in improved productivity and a faster route to market for innovative products and services.
Machine learning excels at analyzing vast datasets for predictive insights, tailored recommendations, and precise anomaly detection, which powers personalized customer experiences, sharper targeting, and more effective resource allocation across sectors from healthcare to finance and e-commerce.
ML-driven automation also delivers direct cost reductions by lowering the need for manual labor, minimizing human errors, and trimming operational expenses such as maintenance, inventory management, and customer service overhead.
Real-world success stories like OpenAI and DeepL showcase how leveraging ML can rapidly scale businesses and create entirely new models for market disruption.
As explained in one founder's experience,
“We grew to 100k/mo visitors in 10 months with AIContentfy.”
The table below illustrates core advantages AI startups can expect from machine learning integration:
Benefit | Description |
---|---|
Efficiency & Automation | Automates routine tasks, freeing up human expertise |
Cost Savings | Reduces manual labor, human error, and operational overhead |
Data-Driven Insights | Analyzes large datasets for better decisions and predictions |
Personalized Experiences | Customizes offerings and boosts customer loyalty |
Scalability | Supports rapid growth without proportional cost increases |
Ultimately, the integration of ML positions your startup to not just compete - but lead - by innovating faster, operating smarter, and delivering greater value than ever before.
For a deeper dive into how automation and predictive analytics can give your business the strategic edge, explore these key concepts and use cases.
Common Challenges for AI Startups Integrating Machine Learning
(Up)Integrating machine learning into AI startup applications brings tremendous opportunities, but it is not without significant hurdles. Startups commonly face issues such as data scarcity, poor data quality, and the high costs associated with acquiring and labeling data, all of which can compromise model accuracy and reliability.
In addition, limitations in skilled talent, infrastructure expenses, and challenges integrating ML models with legacy systems frequently slow down development and deployment.
As noted in a roundup of industry obstacles,
"44% of AI adopters face challenges integrating AI/ML with workflows," and 65% struggle with data quality and accessibility issues, resulting in considerable time and financial strain(common AI/ML implementation challenges for businesses).
Ethical concerns - such as detecting bias, ensuring transparent decision-making, and meeting regulatory requirements - compound these challenges, especially in sectors governed by strict data privacy laws.
Another recurring challenge is the difficulty in scaling solutions:
"Scaling AI pilots to enterprise-wide deployments requires robust architecture and infrastructure, which many organizations lack,"
making it tough for startups to evolve from prototypes to production systems (common barriers to AI adoption and solutions).
Addressing these challenges involves investing in data cleaning and augmentation, leveraging scalable cloud platforms, fostering cross-disciplinary collaboration, and maintaining vigilant compliance with regulations and ethics.
The following table summarizes the most frequent challenges faced by startups:
Challenge Area | Key Details |
---|---|
Data Quality & Access | Scarcity, inconsistency, high cost of quality data |
Talent & Skills | Shortage of ML expertise, difficulty in hiring |
Integration & Infrastructure | Legacy systems, scalability barriers, hardware costs |
Ethics & Regulation | Bias, transparency, regulatory compliance |
By proactively recognizing and planning for these obstacles, startups can craft strategies - such as adopting modular architectures and scalable cloud solutions - to overcome the technical, organizational, and compliance-related bottlenecks of machine learning integration.
For a deeper dive into specific implementation hurdles and proven solutions, see this comprehensive guide to machine learning challenges for startups.
Step-by-Step Guide: How Beginners Can Integrate Machine Learning
(Up)Integrating machine learning into your AI startup application as a beginner involves a structured, collaborative, and goal-driven approach. Start by clearly defining the business problem you intend to solve and outline measurable objectives - involving all relevant stakeholders - to ensure your ML project aligns with your company's strategic vision and delivers true value (aligning AI/ML with business outcomes).
Form a cross-functional team consisting of data scientists, machine learning engineers, data engineers, domain experts, project managers, and ethicists, each playing vital roles, as shown in the table below.
Use inclusive, transparent communication and agile project management to foster team collaboration and continuous learning (guide to building cross-functional AI teams).
Progress through essential phases including data collection and preparation, model development, infrastructure setup, deployment strategy, and ongoing monitoring.
Employ best practices like setting up effective data pipelines, choosing the right tools (e.g., cloud or open-source), establishing key performance indicators (KPIs) for system and user goals, and conducting regular reviews to address challenges such as data bias and evolving business needs (AI/ML startup checklist).
Role | Responsibilities |
---|---|
Data Scientists | Analyze data, develop models, identify patterns |
Machine Learning Engineers | Deploy and scale ML models |
Data Engineers | Design infrastructure, build data pipelines |
Domain Experts | Frame problems, interpret results |
Project Managers | Oversee scope, resources, timelines |
Ethicists/Legal Advisors | Ensure compliance, mitigate risks |
“Clear, understandable goals align team members under a common vision... Goals must be understandable to diverse stakeholders - developers, customers, regulators.”
By following these steps and embracing teamwork, transparency, and ongoing learning, you can lay a strong foundation for integrating machine learning and achieving lasting business impact.
Best Practices and Real-World Tips for AI Startup Success
(Up)Ensuring AI startup success hinges on applying best practices that align technical rigor with business needs and ethical standards. Start by clearly defining your AI and machine learning (ML) strategy in line with measurable business goals and building a solid data foundation through disciplined collection, cleaning, and validation; this is particularly vital for actionable insights and model reliability according to Reenbit's comprehensive guide for IT startups.
Launching small, manageable projects allows teams to iterate rapidly and identify value before scaling, while ongoing collaboration between data science experts, IT, and business teams fosters innovation and integration.
Maintaining data integrity and automation throughout the AI lifecycle streamlines operational overhead - TechCrunch emphasizes vigilant human oversight and the continuous optimization of live, data-driven customer experiences as core to sustainable growth in their review of AI/ML best practices.
Leveraging techniques such as explainable AI strengthens trust and transparency, and establishing continuous feedback loops with users sharpens both model accuracy and user acceptance.
As highlighted in AIContentfy's startup guide, partnerships with AI/ML specialists and an ethical-first mindset are critical - not just to mitigate bias and uphold privacy, but to foster long-term brand trust and differentiate from competitors through responsible innovation.
By incorporating these strategies, your AI startup is better positioned to deliver value, adapt to market changes, and scale effectively.
Advanced Strategies for Scaling Your ML-Powered AI Startup
(Up)Scaling your ML-powered AI startup calls for robust architectural strategies, balancing innovation, speed, and operational resilience. Adopting microservices enables independent scaling, rapid iteration, and improved reliability - critical for handling traffic spikes and minimizing downtime; in fact, migrating from monolithic to microservice architectures helped industry leaders like Netflix, Uber, and Amazon deploy AI capabilities at massive scale, boosting deployment frequency and system resilience (AI microservices scalability case studies).
Equally vital is implementing MLOps practices, which integrate DevOps-style automation, CI/CD pipelines, and containerized deployments - these not only streamline the ML lifecycle but also ensure reproducibility, faster time to market, and transparent governance.
The architecture of modern ML systems increasingly follows a modular pattern: independent pipelines for feature engineering, model training, and inference are connected by a central shared storage layer or feature/model registry (AI modularity and pipeline architecture).
This approach enhances maintainability, allows teams to iterate or scale components separately, and reduces operational bottlenecks as business needs evolve. Startups should also leverage cloud-native patterns: predictive autoscaling driven by ML models boosts efficiency during surges, while infrastructure as code and robust monitoring guard against operational risks and enable rapid global expansion (scalable architecture best practices).
These advanced strategies - when paired with regular model retraining, feature store adoption, and diligent observability - form a resilient foundation that supports sustainable AI innovation and competitive growth.
Frequently Asked Questions
(Up)What are the main benefits of integrating machine learning into an AI startup application?
Integrating machine learning into your AI startup application improves operational efficiency and cost-effectiveness by automating routine tasks, delivers hyper-personalized customer experiences, and unlocks valuable predictive and data-driven insights. This empowers startups to scale rapidly, make better decisions, and maintain a strategic edge over competitors through innovation and smarter operations.
What challenges do AI startups face when integrating machine learning?
Common challenges include data scarcity or poor data quality, high costs for acquiring and labeling data, limited access to skilled talent, integration with legacy systems, infrastructure expenses, and barriers to scalability. Ethical concerns such as model bias, ensuring transparency, and complying with regulations also pose significant challenges for startups.
What is a step-by-step approach for beginners to integrate machine learning in their startup?
Beginners should start by clearly defining the business problem and objectives. Form a cross-functional team including data scientists, ML engineers, data engineers, domain experts, and ethicists. Progress through data collection and preparation, model development, infrastructure setup, deployment planning, and ongoing monitoring. Best practices include leveraging cloud tools, setting KPIs, and iterative reviews to adapt as business needs evolve.
What best practices can help ensure AI startup success when using machine learning?
Successful AI startups define clear, measurable business goals for their ML projects, invest in high-quality data collection and cleaning, and start with small, iterative pilots before scaling. Fostering collaboration among technical and business teams, maintaining vigilant data integrity, leveraging explainable AI, and establishing continuous feedback loops with users are key. Ethical considerations and partnerships with specialists also help build long-term trust.
How can AI startups scale their machine learning solutions effectively?
To scale ML-powered applications, startups should adopt microservices architectures for independent scaling, implement MLOps practices such as CI/CD pipelines and containerization, and use modular system designs. Leveraging cloud-native patterns, autoscaling, infrastructure as code, and robust monitoring enhances resilience and agility. Regular model retraining and adopting feature stores also help sustain reliable and scalable AI systems.
You may be interested in the following topics as well:
Discover how the PostgreSQL flexibility for AI data challenges positions startups to quickly adapt and scale their innovations.
See real-world impacts through E-commerce chatbot solutions that streamline sales and customer interactions in startups.
Stay ahead of vulnerabilities by prioritizing regular updates and patch management for both your AI models and system software.
Stay ahead of the competition by focusing on critical email metrics every AI startup should track for sustainable growth.
See why TransPerfect's global network is a trusted powerhouse for enterprise-grade localization in over 200 languages.
Acknowledge the challenges unique to solo AI founders and learn how to tackle them head-on.
Explore our detailed cost comparison: self-hosting vs. cloud AI services to see which model better aligns with your budget and growth plans.
Unlock the secrets of testing core assumptions with data to minimize risk before you commit resources.
Ludo Fourrage
Founder and CEO
Ludovic (Ludo) Fourrage is an education industry veteran, named in 2017 as a Learning Technology Leader by Training Magazine. Before founding Nucamp, Ludo spent 18 years at Microsoft where he led innovation in the learning space. As the Senior Director of Digital Learning at this same company, Ludo led the development of the first of its kind 'YouTube for the Enterprise'. More recently, he delivered one of the most successful Corporate MOOC programs in partnership with top business schools and consulting organizations, i.e. INSEAD, Wharton, London Business School, and Accenture, to name a few. With the belief that the right education for everyone is an achievable goal, Ludo leads the nucamp team in the quest to make quality education accessible