Understanding Data Privacy Laws for Solo AI Startups Across Different Regions
Last Updated: May 22nd 2025
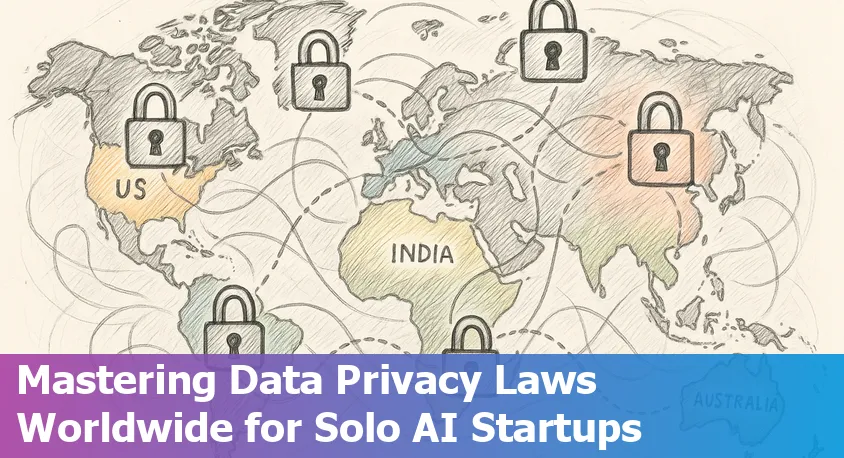
Too Long; Didn't Read:
Solo AI startups must navigate a complex global data privacy landscape, with strict regulations like GDPR in Europe, CCPA in California, and PIPL in China. Key compliance steps include data minimization, privacy-by-design, and localization solutions. Prioritizing transparency and ethical AI builds trust and reduces the risk of fines - up to €20M or 4% global turnover under GDPR.
As a solo AI startup founder looking to expand globally, understanding data privacy is not just a legal necessity - it's a strategic imperative. AI's reliance on vast, sensitive datasets introduces complex risks such as unauthorized data use, covert data collection, algorithmic bias, and difficulties in complying with a mosaic of international privacy regulations.
For more details, see the DataGuard Insights on AI privacy challenges.
Multiple regions take diverse approaches: Europe enforces strict data rights under GDPR, while the U.S. landscape remains fragmented, with state-level laws and no sweeping federal equivalent - making unified compliance a significant operational challenge.
This is explored by CSIS in their AI data privacy analysis.
For founders, prioritizing privacy from day one strengthens stakeholder trust, enhances brand reputation, and differentiates your AI solution in a marketplace where “privacy by design” is increasingly expected.
See Forbes on privacy as a strategic advantage.
As this blog explores, navigating these shifting expectations and laws requires vigilance, transparency, and a global mindset - keys for solo entrepreneurs aiming to not just comply, but thrive in the age of AI.
Table of Contents
- What Makes AI Data Privacy Unique Across Multiple Regions?
- Key Data Privacy Laws Around the World Every Solo AI Startup Must Know
- Data Localization Challenges for Solo AI Startups: Practical Solutions Across Jurisdictions
- Best Practices for Privacy Compliance as a Solo AI Startup Across Regions
- Common Pitfalls and Risk Areas in Cross-Border AI Data Practices
- Building Trust: Meeting Consumer Expectations for AI Privacy Across Different Regions
- Conclusion: Setting Up Solo AI Startups for Privacy Success Worldwide
- Frequently Asked Questions
Check out next:
Discover why AI startup opportunities in 2025 are more accessible than ever for solo founders ready to make a global impact.
What Makes AI Data Privacy Unique Across Multiple Regions?
(Up)AI data privacy challenges differ significantly across regions due to the sheer scale, complexity, and automation inherent in modern artificial intelligence technologies.
Unlike traditional data systems, AI models often require vast, diverse datasets and employ opaque “black box” decision-making, amplifying concerns about bias, explainability, and compliance in disparate global jurisdictions.
As the Office of the Victorian Information Commissioner explains the privacy challenges of AI, “AI could also enhance privacy by reducing human access to raw data and enabling personalized consent,” but simultaneously complicates core privacy principles, informed consent, and the very definition of “personal information.” AI-specific challenges such as model vulnerability to data breaches, reidentification, and discrimination are compounded when operating across varying legal frameworks, including Europe's GDPR, the U.S.'s patchwork of state laws, and emerging standards like the EU AI Act.
For solo AI startups, it is essential to bridge the “transparency gap” and proactively address local requirements - including consent management, algorithmic accountability, and cross-border data transfer restrictions - since many countries are now expanding their regulatory expectations and enforcement.
As highlighted in VeraSafe's analysis of privacy concerns in AI, the right to opt out, right to explanation, and the difficulty of deleting deeply embedded personal data in large language models are just a few of the technical and regulatory hurdles to overcome.
Furthermore, as summarized by Secure Privacy's 2025 AI GDPR compliance review, the intersection of AI and multi-region privacy compliance demands robust strategies: data minimization, privacy impact assessments, and technical documentation are mandatory to avoid steep penalties and reputational damage while earning consumer trust worldwide.
Key Data Privacy Laws Around the World Every Solo AI Startup Must Know
(Up)Navigating international data privacy laws is foundational for solo AI startups aiming to operate across borders. Key regulations include the European Union's General Data Protection Regulation (GDPR), California's Consumer Privacy Act (CCPA) and its updated CPRA, Brazil's Lei Geral de Proteção de Dados (LGPD), Canada's Personal Information Protection and Electronic Documents Act (PIPEDA), and China's Personal Information Protection Law (PIPL) - each establishing unique rights and obligations for processing personal data.
The GDPR stands out with its extraterritorial reach and strict requirements - explicit consent, quick breach notification (within 72 hours), and broad rights for individuals such as access, deletion, and objection to processing - enforced through fines up to €20 million or 4% of global turnover.
CCPA and CPRA, effective in the US, mandate transparency, opt-out mechanisms for data sale, as well as expanded rights around sensitive data and rectification, enforced by the California Privacy Protection Agency.
LGPD, closely modeled after GDPR, requires a legal basis for data use and empowers Brazil's national data protection authority (ANPD) to enforce individual data correction, deletion, and transfer.
The variety and evolving nature of these laws demand tailored compliance strategies; for example, PIPEDA emphasizes consent and ongoing breach reporting for Canadian users, while China's PIPL sets some of the strictest global consent and penalty frameworks.
The table below highlights several parallels and distinctions among these major privacy regimes:
Law | Key Coverage Area | Notable Individual Rights | Max Penalties |
---|---|---|---|
GDPR (EU) | All personal data of EU residents | Access, correction, deletion, objection, portability | €20M or 4% global turnover |
CCPA/CPRA (California) | Personal data of California residents | Access, deletion, opt-out, correction (CPRA), limit use of sensitive data | $7,500 per violation |
LGPD (Brazil) | Personal data processed in Brazil | Access, rectification, deletion, portability, information on third-party data sharing | 2% of Brazilian revenue |
PIPL (China) | Data on Chinese citizens worldwide | Access, copy, correction, deletion, withdrawal of consent | 5% previous year's revenue |
Data Localization Challenges for Solo AI Startups: Practical Solutions Across Jurisdictions
(Up)Solo AI startups confronting data localization laws face formidable challenges in navigating conflicting requirements across jurisdictions, particularly as regulations tighten in 2025.
The new U.S. Department of Justice rule, taking effect April 8, 2025, strictly prohibits certain cross-border data transfers - especially those involving sensitive personal data categories such as biometric information, health records, and human 'omic data - to designated “Countries of Concern,” with steep penalties for non-compliance and additional mandates for contractual safeguards and CISA-approved security controls US Data Localization Law Coming Soon: DOJ Issues Final Rule.
Meanwhile, Europe's GDPR prioritizes stringent privacy controls, China's PIPL requires domestic data storage for resident data, and recent EU and APAC sector regulations add further complexity AI data residency regulations and challenges.
To practically manage these burdens, industry experts recommend leveraging distributed or hybrid infrastructure - keeping regulated data within required borders, adopting consent-driven cross-border solutions, encrypting data at rest and in transit, and favoring cloud providers with regional hosting options.
As the Equinix report notes, Knowing the exact location of your data is critical for compliance,
and federated AI solutions - where models are trained locally or edge-side with only anonymized weights shared - enable innovation without contravening data residency rules.
Practical measures like these not only reduce legal risk but help solo founders maintain agility and build trust in their AI offerings Data Sovereignty and AI: Why You Need Distributed Infrastructure.
The table below outlines key bulk data thresholds under the new U.S. rule:
Data Type | Bulk Threshold (U.S. Persons/Devices) |
---|---|
Human ‘Omic Data | 1,000 persons; genomic data > 100 persons |
Biometric Identifiers | 1,000 persons |
Precise Geolocation Data | 1,000 devices |
Personal Health Data | 10,000 persons |
Personal Financial Data | 10,000 persons |
Covered Personal Identifiers | 100,000 persons |
AI relies heavily on data, raising concerns about where data is stored, processed, and accessed. Compliance with geographical legal and regulatory requirements for data is critical while balancing innovation.
Best Practices for Privacy Compliance as a Solo AI Startup Across Regions
(Up)For solo AI startups operating across regions, privacy compliance hinges on adopting a combination of best practices that address both regulatory requirements and evolving technological demands.
Start with data minimization - limit data collection and retention to only what is essential for your model's objectives to reduce risks and ease cross-border compliance pressures, as enforcement of minimization principles now extends from the EU's GDPR to U.S. laws like the CCPA (global data minimization requirements).
Implement privacy-by-design strategies throughout your AI lifecycle: conduct regular privacy impact assessments, use anonymization and differential privacy for datasets, and ensure all user consent is clear, granular, and revocable.
As summarized by privacy experts,
“create a data map to identify where critical and sensitive information is stored,”
leverage federated learning or privacy-enhancing technologies to train models without pooling raw data centrally, and foster a culture prioritizing privacy awareness (tested data minimization strategies for startups).
Document and review your compliance frameworks to demonstrate transparency, and engage with regulators proactively to anticipate future requirements (strategies for compliance and innovation in AI data privacy).
Structured disciplined privacy practices not only reduce legal exposure but also build consumer trust - setting the foundation for ethical growth in global AI markets.
Common Pitfalls and Risk Areas in Cross-Border AI Data Practices
(Up)Navigating cross-border AI data practices exposes solo startups to a network of common pitfalls and risk areas, including strict data localization policies, varied regulatory demands, and privacy challenges intrinsic to AI itself.
A key challenge is complying with evolving data localization measures, which have more than doubled globally from 67 policies in 2017 to 144 in 2021 - significantly raising operating costs, undermining productivity, and reducing opportunities for innovation and trade, as outlined in this analysis of the expanding costs and consequences of cross-border data flow barriers.
The complexity is further compounded by the technological aspect of AI, where pitfalls such as unauthorized or covert data collection, algorithmic bias, and ambiguous consent mechanisms can lead to breaches, operational disruption, and regulatory fines, especially as frameworks like GDPR, CCPA, and HIPAA enforce strong user protections - details covered in best practices for mitigating generative AI privacy risks.
Additionally, emerging trends in explainable AI, privacy-enhancing computation, and the need for differentiated regulation based on data type (raw, intermediary, synthetic) are critical for startups to consider; as noted,
“Transparency builds trust by openly communicating how data is collected, used, and transferred.”
For practical solutions and a broader regulatory landscape, startups should also familiarize themselves with global compliance strategies, secure transfer technologies, and the importance of agility as described in this resource for navigating cross-border AI data transfer challenges.
Risk Area | Description |
---|---|
Data Localization | Mandatory data storage rules, increasing compliance costs and legal risks |
AI Bias/Transparency | Potential for discriminatory outcomes and opaque decision processes |
Informed Consent | Difficulties providing clear, actionable user consent due to AI complexity |
Building Trust: Meeting Consumer Expectations for AI Privacy Across Different Regions
(Up)Building trust is core for solo AI startups navigating the complexities of cross-regional data privacy - especially as consumer expectations surge worldwide. Recent studies consistently show that consumers demand ethical AI, robust transparency, and real control over their personal data.
According to Forbes' summary of Cisco's 2024 Consumer Privacy Survey, 78% of respondents expect companies to commit to ethical AI standards, and over 80% would feel more comfortable with AI-powered products if clear transparency and bias audits are in place - signaling that privacy is now a customer requirement, not just a regulation checkbox.
Echoing these findings, Deloitte's Connected Consumer survey highlights that 90% of people believe tech companies must do more to protect their data, with 79% finding privacy policies unclear, and 52% reporting high trust only when policies are transparent and controls are easy to manage.
As articulated in TrustCloud's best practices for ethical AI,
“Balancing AI's power and data privacy requires ethical frameworks and proactive measures,”
pinpointing practices such as privacy-by-design, regular audits, and continuous customer communication as vital to fulfilling these expectations (ethical considerations for AI privacy).
This trend is more than personal sentiment; business impact is clear - organizations that establish trust through transparent data policies and privacy investments outperform those that don't.
The following table highlights key consumer attitudes in 2024:
Metric | Stat |
---|---|
Desire for ethical AI standards | 78% |
Comfort with AI after transparency & ethics ensured | >80% |
Trust tech companies to protect data | Only 21% some/great trust (Pew); majority want more regulatory action |
For solo AI founders, embracing transparency, ethical frameworks, and clear customer communication is not just regulatory best practice - it is the cornerstone of lasting trust and successful AI adoption in every region (Deloitte's survey on consumer trust and AI privacy).
Conclusion: Setting Up Solo AI Startups for Privacy Success Worldwide
(Up)Setting up a solo AI startup for privacy success worldwide means actively embedding transparent, ethical, and regionally-compliant data practices from day one.
As global regulations like GDPR, CCPA, and the emerging EU AI Act evolve, founders must employ a “privacy by design” mindset - minimizing data collection, securing sensitive information, and preserving user rights throughout the AI lifecycle.
Regulatory experts emphasize that robust governance, regular audits, and accountability are essential to sustain trust and reduce risk, while leading privacy summits highlight that harmonizing compliance frameworks across jurisdictions fosters both innovation and resilience (Key Takeaways from the IAPP Global Privacy Summit 2025).
For sole founders reliant on third-party AI vendors, due diligence, contract clarity, and ongoing oversight remain non-negotiable, with recent case studies illustrating that
“organizations remain legally responsible for compliance with privacy laws, even when outsourcing to AI vendors” - risking steep penalties for lapses(VeraSafe: AI Vendors and Data Privacy Essential Insights for Organizations).
As enforcement ramps up and complex data flows become standard, best-in-class solo startups will establish adaptable privacy frameworks, stay proactive with training and monitoring, and champion responsible AI as a business advantage.
Ultimately, privacy stewardship is not just about compliance - it's a springboard for building sustainable, trusted global AI ventures that can confidently scale across regions.
Frequently Asked Questions
(Up)What are the major data privacy laws that solo AI startups must consider in different regions?
Solo AI startups operating globally must navigate a variety of key data privacy laws, including the European Union's GDPR, California's CCPA and CPRA, Brazil's LGPD, Canada's PIPEDA, and China's PIPL. Each law establishes specific user rights (such as access, deletion, and consent), obligations for handling data, and penalties for non-compliance, with some - like GDPR and PIPL - having extraterritorial reach and steep fines.
What makes AI data privacy uniquely challenging across different jurisdictions?
AI data privacy is uniquely complex due to AI's reliance on vast, sensitive datasets, the opacity of AI models, and the automation of decision-making. This creates challenges in achieving transparency, managing algorithmic bias, and ensuring informed user consent, all while adhering to diverse regional legal frameworks such as GDPR and CCPA. Solo AI founders must address local consent rules, cross-border transfer restrictions, and growing expectations for algorithmic explainability.
How can solo AI startups handle data localization and cross-border data transfer regulations?
To comply with data localization and cross-border regulations, solo AI startups should use distributed or hybrid cloud infrastructure to keep data where required, rely on regional hosting providers, encrypt data in transit and at rest, and explore federated learning techniques. Staying updated on regional rules - like the US Department of Justice's 2025 bulk data transfer thresholds and China's strict PIPL localization - is critical to avoid penalties.
What are recommended best practices for privacy compliance as a solo AI startup?
Best practices include adopting privacy-by-design from the outset, minimizing data collection and retention, conducting regular privacy impact assessments, using anonymization or privacy-enhancing techniques, maintaining clear, revocable consent mechanisms, and documenting compliance processes. Startups should also proactively communicate with regulators and prioritize transparency to foster user trust.
What are common pitfalls for solo AI startups handling data across borders and how can they build trust?
Solo AI startups often stumble over strict localization rules, evolving regulations, unclear user consent, and algorithmic bias. To build trust, startups should emphasize transparency, ethical data handling, and regular audits - aligning their privacy strategy with global consumer demands for control and ethical AI. Demonstrating clear data practices and responsive communication helps meet both legal requirements and rising stakeholder expectations for privacy.
You may be interested in the following topics as well:
Explore how data privacy and regulatory compliance can shape the foundation of your solo AI startup journey.
Stay ahead of the curve using Scrut's always-on multi-cloud compliance for complete visibility across your AI stack.
Experience the convenience of virtual legal assistant support for 24/7 communication, lead screening, and appointment management.
Get a breakdown of the best hosting providers for AI startups and what they offer in terms of speed, support, and costs.
Jumpstart your analytics journey with no-code AI tools for solo founders, making advanced technology accessible even to non-technical entrepreneurs.
Level up your workflows with self-hosted automation plugins for AI startups that rival SaaS competitors - minus the ongoing fees.
See why Synthesia video creation is revolutionizing onboarding and marketing with accessible, AI-powered videos.
Unlock the potential of your AI startup by understanding the self-hosting benefits for solo AI founders.
Ludo Fourrage
Founder and CEO
Ludovic (Ludo) Fourrage is an education industry veteran, named in 2017 as a Learning Technology Leader by Training Magazine. Before founding Nucamp, Ludo spent 18 years at Microsoft where he led innovation in the learning space. As the Senior Director of Digital Learning at this same company, Ludo led the development of the first of its kind 'YouTube for the Enterprise'. More recently, he delivered one of the most successful Corporate MOOC programs in partnership with top business schools and consulting organizations, i.e. INSEAD, Wharton, London Business School, and Accenture, to name a few. With the belief that the right education for everyone is an achievable goal, Ludo leads the nucamp team in the quest to make quality education accessible